Automated biofoundries and smart biomanufacturing
We boost biomanufacturing technologies
Our group is dedicated to advancing the potential of synthetic biology in providing sustainable manufacturing processes that reduce reliance on fossil fuels and contribute to an environmentally friendly economy.
We focus on intelligent biodesign automation and innovative biomanufacturing technologies, working to minimize scale-up costs and times. First, we generate designs automatically by providing a list of genetic parts to be synthesized. The construction and testing phases are carried out by robotic platforms for full traceability. Experimental results are then fed back into learning and design engines, streamlining the process, and promoting a profitable, sustainable, and efficient approach to biomanufacturing.
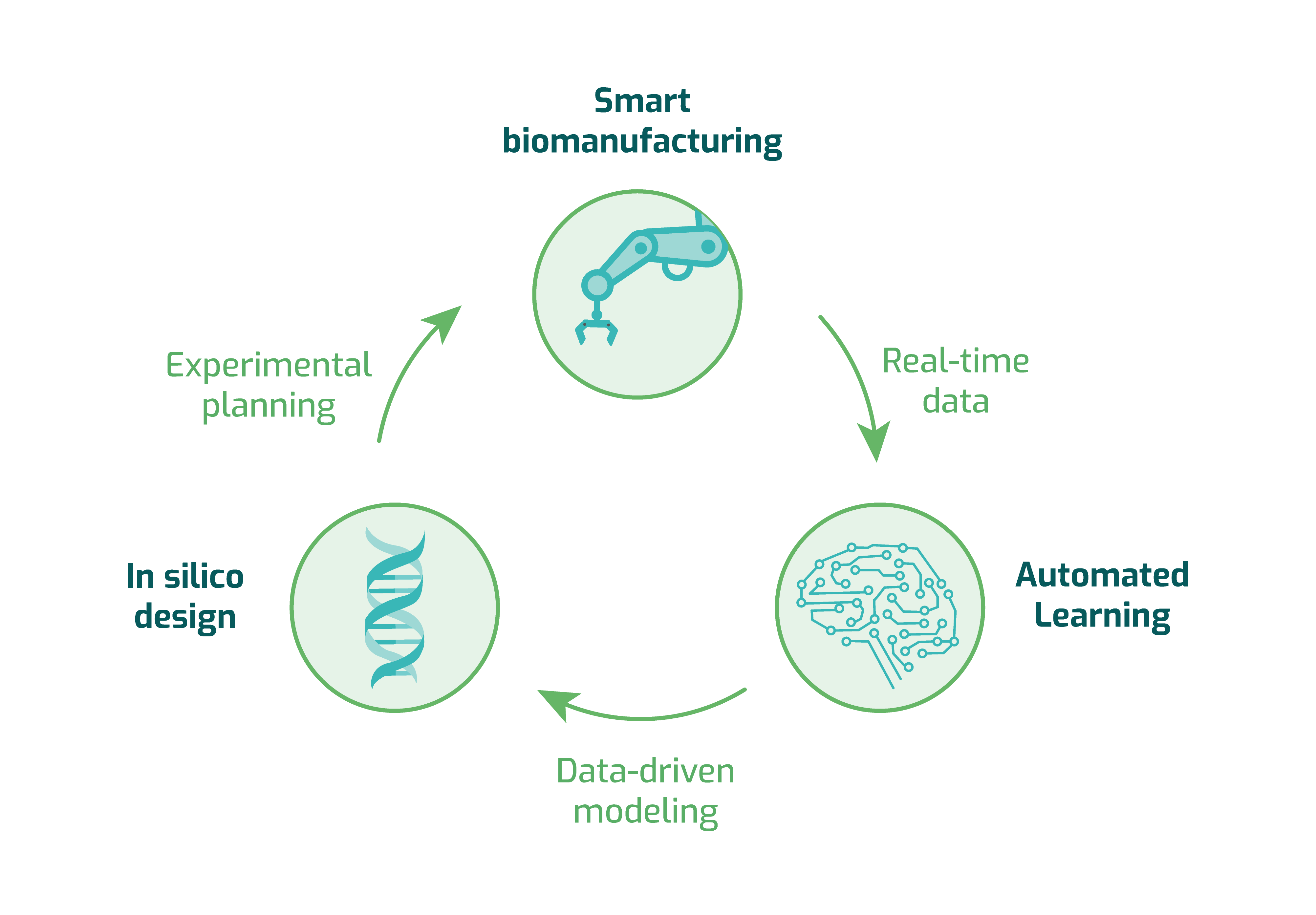
Automated biofoundries and smart biomanufacturing
We boost biomanufacturing technologies
Our group is dedicated to advancing the potential of synthetic biology in providing sustainable manufacturing processes that reduce reliance on fossil fuels and contribute to an environmentally friendly economy.
We focus on intelligent biodesign automation and innovative biomanufacturing technologies, working to minimize scale-up costs and times. First, we generate designs automatically by providing a list of genetic parts to be synthesized. The construction and testing phases are carried out by robotic platforms for full traceability. Experimental results are then fed back into learning and design engines, streamlining the process, and promoting a profitable, sustainable, and efficient approach to biomanufacturing.
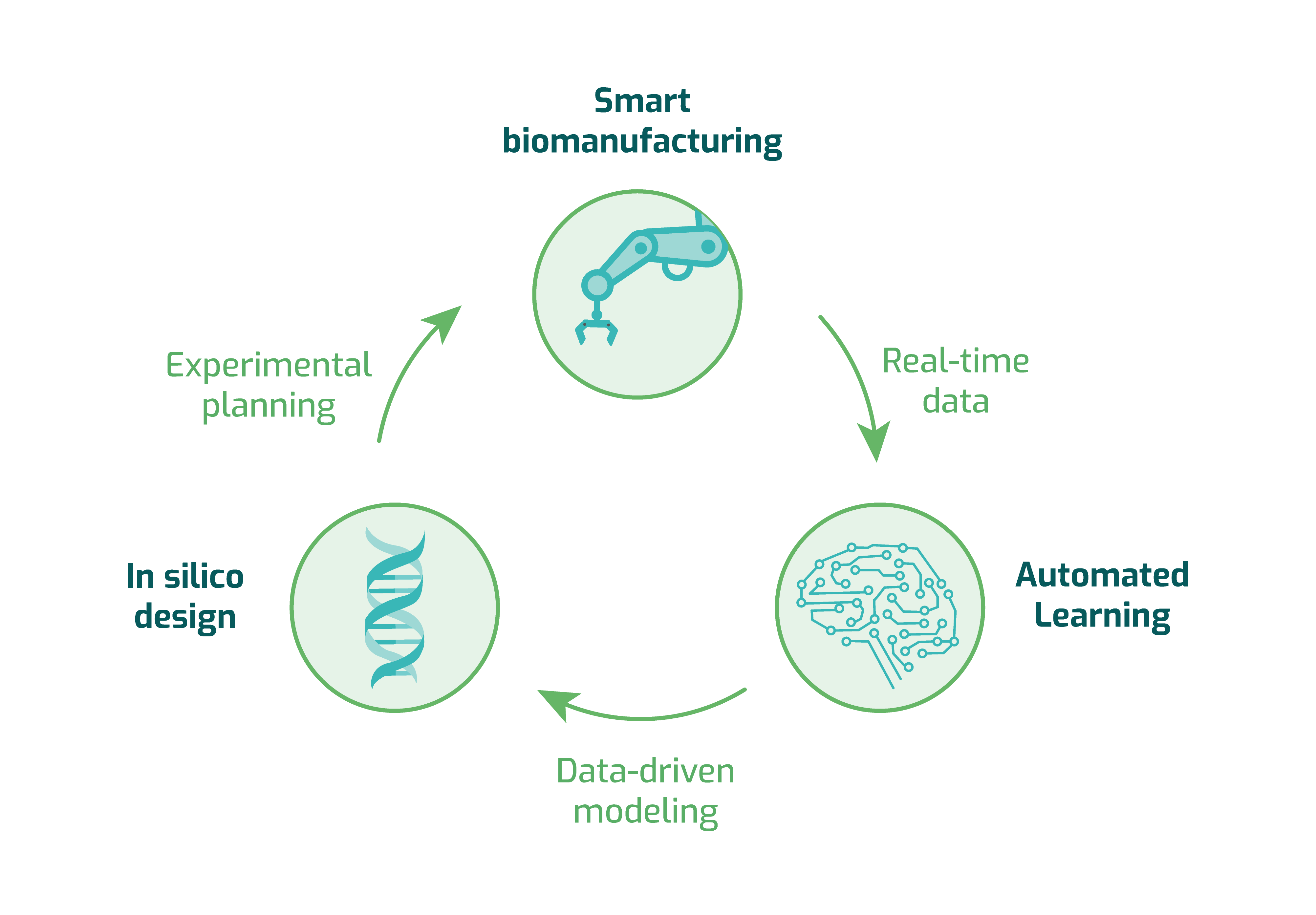
Our research lines
Advancing synthetic biology
AI-based genetic circuit design
Enhancing bioengineering precision with machine learning
We explore the integration of machine learning and automation into the synthetic biology Design-Build-Test-Learn (DBTL) cycle. By leveraging machine learning techniques such as deep learning and Gaussian processes, we optimize genetic circuit design and improve enzyme and biosensor design. Our goal is to achieve a more precise and predictable bioengineering process.
Extended metabolic space
Uncovering novel biosynthesis pathways
We combine systems biology, machine learning, and graph theory to model and predict enzyme promiscuity and create innovative metabolic engineering solutions. By modeling these promiscuous interactions, we aim to identify alternative biosynthesis pathways for therapeutics and to evaluate small compound toxicity induced by such interactions. Our methodology has led to the creation of the first enzyme promiscuity predictor with over 80% accuracy and the development of valuable tools such as RetroPath/XTMS, which are widely used by the metabolic engineering community.
Synthetic biology and biomanufacturing automation in biofoundries
Streamlining metabolic engineering
We contribute to the development of design tools for metabolic engineering within the Design – Build – Test – Learn cycle, such as RetroPath2.0 and Selenzime. By integrating these tools, we create a seamless process to optimise microbial strains for the production of fine and specialty chemicals. Thus, we improve efficiency and expand natural chemical diversity.
Our research lines
Advancing synthetic biology
AI-based genetic circuit design
Enhancing bioengineering precision with machine learning
We explore the integration of machine learning and automation into the synthetic biology Design-Build-Test-Learn (DBTL) cycle. By leveraging machine learning techniques such as deep learning and Gaussian processes, we optimize genetic circuit design and improve enzyme and biosensor design. Our goal is to achieve a more precise and predictable bioengineering process.
Extended metabolic space
Uncovering novel biosynthesis pathways
We combine systems biology, machine learning, and graph theory to model and predict enzyme promiscuity and create innovative metabolic engineering solutions. By modeling these promiscuous interactions, we aim to identify alternative biosynthesis pathways for therapeutics and to evaluate small compound toxicity induced by such interactions. Our methodology has led to the creation of the first enzyme promiscuity predictor with over 80% accuracy and the development of valuable tools such as RetroPath/XTMS, which are widely used by the metabolic engineering community.
Synthetic biology and biomanufacturing automation in biofoundries
Streamlining metabolic engineering
We contribute to the development of design tools for metabolic engineering within the Design – Build – Test – Learn cycle, such as RetroPath2.0 and Selenzime. By integrating these tools, we create a seamless process to optimise microbial strains for the production of fine and specialty chemicals. Thus, we improve efficiency and expand natural chemical diversity.