Exploring the extended metabolic space.
Our group has proposed machine learning modeling techniques for precise control of complex nonlinear dynamics to highly interconnected systems such as biological networks. In PLoS Comp Biol, 2007, we introduced a large-scale systems biology analysis of protein domains. The proposed model predicted the role of binding residues determining promiscuity from a combination of the information on interacting molecular structures, that were coded using graph-based representations, machine learning, and systems biology characteristics from protein interaction databases.
At the Institute of Systems and Synthetic Biology (Univ. Paris-Saclay/Genopole and CNRS in France), we applied systems biology and machine learning modeling principles for enzyme promiscuity to metabolic engineering. The objective was to model promiscuous interactions to identify alternative biosynthesis pathways for therapeutics and to evaluate small compound toxicity induced by such promiscuous interactions. Our innovative approach combines:
- 1
Encoding the space of biochemical states of the cell by means of a graph representation of molecular signatures of the known biochemical transformations;
- 2
Integration of that representation with the analysis of the cell’s metabolic state space by means of stochiometric matrices (genome-scale models);
- 3
Development of design techniques in the metabolic state space extended by pathway enumeration;
- 4
Use of machine learning approaches for the prediction of enzymes, production routes, biosensors, and biological activities such as substrate affinity or toxicity (Bioinformatics, 2010).

The proposed methodology was based on the application of graph theory and machine learning to code and predict biochemical reactions that are catalyzed by promiscuous enzymes (Bioinformatics, 2010) achieving accuracy above 80%. This was the first enzyme promiscuity predictor available in the literature, which can be used to identify residues associated with specific reactions and to propose mutations that can modulate the desired catalytic activity.
Our promiscuity prediction approach was applied to retrosynthesis in metabolic engineering for pathway design, a methodology originally proposed for synthetic chemistry based on a backward search of enzymatic steps connecting the target product to the host organism (BMC Sys Biol, 2011)3. Putative biosynthesis pathways can be identified by this approach, enumerated, and ranked through a multi-parameter optimization, such as gene compatibility, steady-state fluxes and predicted metabolite toxicity (Figure 2). We developed several tools, RetroPath/XTMS (ACS Synth Biol, 2014; Nucleic Acids Res,2014), that are routinely applied and accessed by the metabolic engineering community.
A) Both biochemical reactions and macromolecular structures like proteins can be represented by means of a decomposition into graph-base elementary descriptors. By these means, a metabolic route can be represented as a interconnection circuit of molecular descriptors.
B) From the point of view of biosynthetic production, it is possible to design circuits for actuation, biosensing, regulation, and signal processing.
C) Metabolic circuits design can be framed as a multiobjective optimization problem.
D) Experimental validation of the objective function in a case of polyphenol production including reactions R fluxes v and compounds C.
E) Example of enumeration of design solutions for the metabolic production of flavonoids in bacteria.
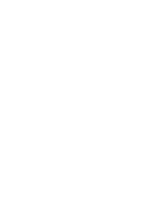