AI-based genetic circuit design
The bio-based production of chemicals and materials is anticipated to play a major role in the incoming bioeconomy by providing an alternative to chemical processes that is both economically viable and ecologically sustainable. The synthetic biology market is expected to reach beyond 40 billion dollars by 2030 globally. In the new biomanufacturing pipelines, modeling, automation, machine learning and big data analysis are becoming essential in order to optimize the processes. Based on this vision, our research interests have been focused on fostering the role of machine learning and engineering as major enabling technologies for leveraging synthetic biology and biomanufacturing.
Machine learning and automation can be used to improve the synthetic biology Design-Build-Test-Learn (DBTL) cycle in different ways. Automation allows rapid growing and assembly of genetic designs through robotics and microfluidics platforms, high-throughput omics quantification, and experimental data analysis. Machine learning can drive each step in the cycle through generation of the experimental planning, smart selection of samples for quantification, model inference from experimental data and design rules for the next iteration. Examples of competing objectives and preliminary results are represented for each step of the cycle.
The full automation of processes in the design-build-test-learn synthetic biology pipeline is currently facilitating the engineering of synthetic parts and circuits in a high-throughput fashion generating large amounts of data. There are multiple opportunities and scenarios where machine learning-based solutions can be integrated as part of that pipeline: machine learning can be applied in order to infer design rules for synthetic biology parts, systems and devices including prediction of sequence-activity relationships like transcriptional (promoter design), translational (ribosome-binding site tuning) and post-translational regulation; enzyme design for increased substrate affinity, catalytic efficiency or to develop novel activities through directed evolution; as well as on the design of other specialized activities such as biosensors or transporters.
However, synthetic parts and circuits do not necessarily behave as planned and the engineering process is hampered by failures. The complexity associated with the large combinatorial design space is a major challenge, i.e., the number of candidate enzymes and regulatory elements for typical bioproduction pathways has a combinatorial complexity of possible designs that cannot be fully explored even on robotized platforms. Our group focuses on addressing key, but largely unexplored complexity problems associated with metabolic pathway and synthetic biology design. The hypothesis is that rationalizing the approach to genetic circuit design by following strategies like those in manufacturing engineering design will lead to a more precise and predictable bioengineering.

Main approaches:
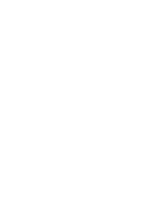